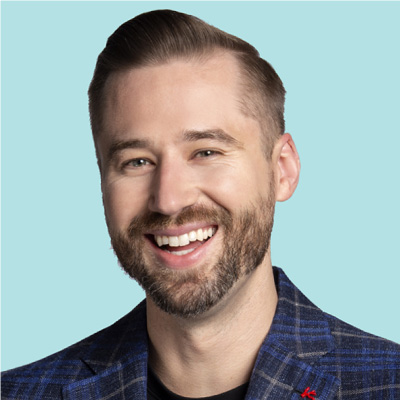
As CEO and founder of Absci (NASDAQ:ABSI), a drug and target discovery company harnessing deep learning AI and synthetic biology to expand the therapeutic potential of proteins, Sean McClain has come a long way in the past 10 years. With a vision to revolutionize the way protein-based drugs are developed, Mr. McClain, a biochemist and molecular biologist, founded Absci in a tiny basement lab complete with surplus second-hand equipment. That’s in stark comparison to where Absci, which went public in July 2021, is now: a leader in AI and synthetic biology with more than 200 employees headquartered at its own campus in Vancouver, WA. In this interview with BioTuesdays, Mr. McClain discusses how Absci is transforming biologic drug discovery with the benefits of deep learning and synthetic biology.
Let’s begin with a brief history of Absci.
In 2011, my goal was to do something many thought was impossible — to use DNA to program E. coli bacteria to make the next generation of protein-based drugs. Not only have we been able to reach that goal, but we have since grown into a company that is redefining the paradigm of drug discovery and biomanufacturing, merging artificial intelligence with synthetic biology to get the best possible drugs to patients with unprecedented speed. Our mission is to change the world one protein at a time.
How did you arrive at using DNA to program E. coli bacteria to make the next generation of protein-based drugs?
When I was at the University of Arizona, I came up with this idea of engineering E. coli to be more mammalian-like to produce complex therapeutic proteins, and this became the basis of Absci. I graduated a year early, moved back in with my parents and began developing this proof of concept. This technology is still fundamental to where we are today as we’ve continued to evolve.
Can you explain your catch phrase, “We translate ideas into drugs.”?
We’re on the forefront of merging AI and biology together to ultimately get better drugs to patients. To translate ideas into drugs involves taking our partners’ ‘wish list’ for drug discovery and applying our platform and technology to make that a reality in a way that hasn’t been done before. Because we’re leveraging AI, it allows us to search the universe of possible drug candidates and precisely pick out the most promising versions to test in the lab for a given target or indication. Also, because our discovery efforts are focused on therapeutic proteins, which have to be made with cellular machinery in a process called translation, the catch phrase is also a bit of a play on words for biology nerds like us.
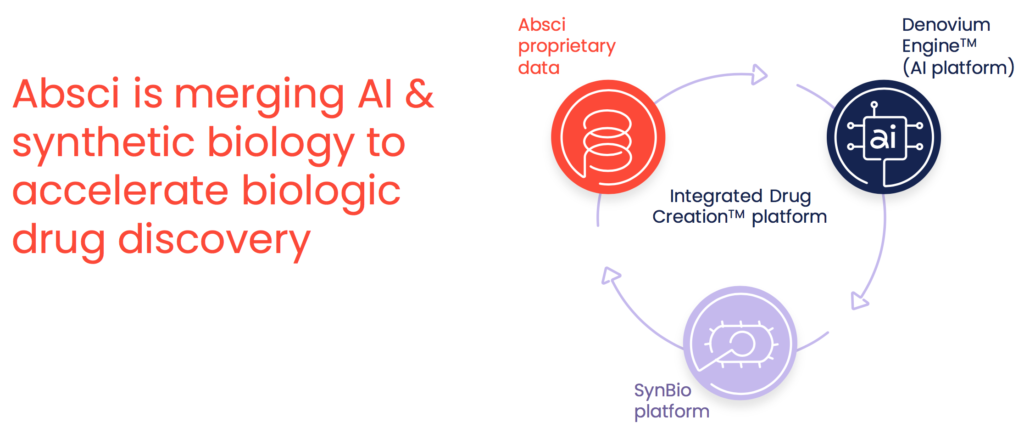
Can you explain how you merge AI and synthetic biology to accelerate biologic drug discovery?
We are a data-centric company and have built our Integrated Drug Creation Platform to identify novel drug targets, discover optimal biotherapeutic candidates and generate the cell lines to manufacture them in a single efficient process. But biologic drug discovery is a complex combinatorial challenge because the number of potential monoclonal antibody sequences exceeds the total number of atoms in the universe, and the overwhelming majority of these potential sequences could never make a promising drug candidate. Even with our breakthrough high-throughput assays that we’ve developed on our synthetic biology platform, we can’t possibly screen all the variations. For the variants we do screen, however, we generate large datasets about drug candidates’ affinities for targets and other developability and manufacturability parameters. These datasets are the perfect substrate for AI.
So that’s where it all comes together. We use the synthetic biology assay data to train cutting edge AI models that can then help us sort through this immense sequence space in silico. That way, when it comes to selecting which sequences to test in the lab, we are taking shots on goal instead of making blind passes. So enriching our starting set for sequences that have the best potential as drug candidates is a promising way to drive efficiency and improve the drug discovery process. And our AI is getting better and better as we continue to feed it more data from our assays. We have deep contextual language AI models that can generate antibodies with specified target affinity. We’ve used Al functional embeddings to identify novel chaperones that improve drug candidate manufacturability. And alongside all that, with our non-natural amino acid incorporation technology we create what we call “Bionic Proteins” that allow us to out-evolve nature and design drug candidates with custom chemistry.
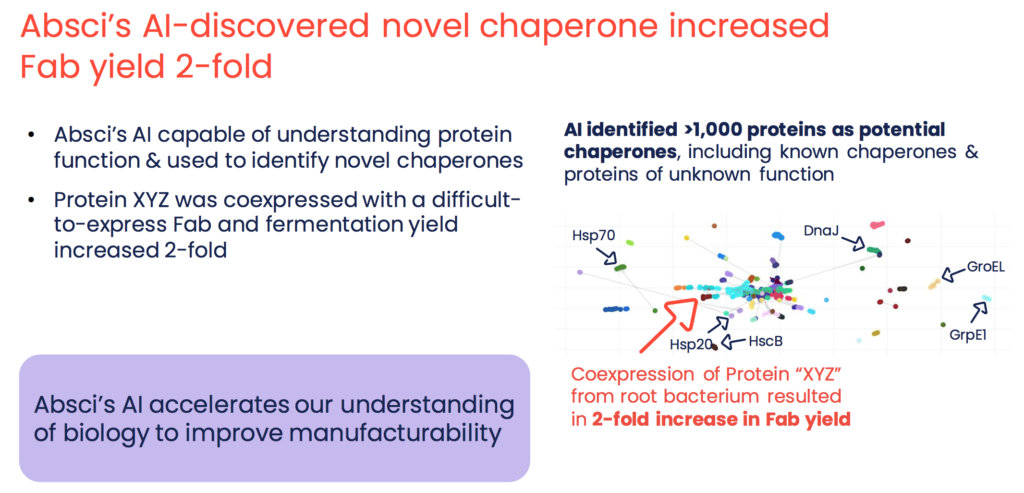
Why does conventional biologic drug discovery often fail?
Existing technologies have about a 4% success rate, which I believe stems from the fact that their protein scaffolds are biologically foreign, which presents challenges for conventional biologic discovery and cell line development. These methods typically involve a series of disjointed steps to screen and select desired molecular parts and reformat them into their final protein scaffold, with subsequent laborious and often unsuccessful generation of a suitable manufacturing cell line. And those are just the discovery hurdles – preclinical developability challenges that require lead optimization may necessitate a return to the drawing board, or worse, cause a clinical trial failure later in development. We are building our platform to apply our AI-driven synthetic biology approach to create lead drug candidates that have been designed in silico for optimal developability and other desired characteristics. We create these proteins in the desired scaffold format (monoclonal antibody or next-generation modality) and in the scalable production cell line. So instead of a disjointed stepwise process, ours is an end-to-end integrated solution purpose-built to reduce multiple failure points and improve drug development success.
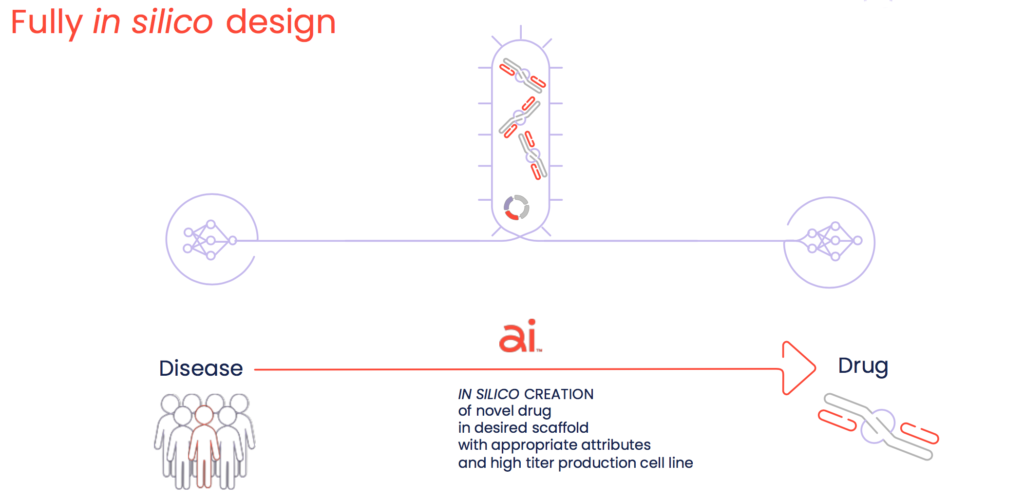
Can you discuss your discovery and cell line development categories?
We define discovery as any projects where we are evaluating variants of the protein of interest, and we define cell line development as a program for which the production cell line alone is the goal of a partnership. It’s really whatever the partner needs at whatever stage the partner is at. We believe our approach delivers disruptive efficiency, but more importantly enables us to create for our partners novel human- and AI-designed next-generation biologics.
How many active drug programs do you have underway?
We have 15 Active Programs and have guided to five additional new Active Programs in 2022, the majority of which we expect will be for drug discovery.
How have you financed Absci?
From the date of our formation up to our IPO in July 2021, we raised $230-million primarily through private placements. We raised an additional $230-million in the IPO.
Can you run through the elements of your Merck collaboration?
Absci will deploy its Bionic Protein non-standard amino acid technology to produce enzymes tailored to Merck’s biomanufacturing applications and we will receive an upfront and certain other milestone payments. In addition, Merck has the option to nominate up to three targets and enter into a drug discovery agreement. Absci would then be eligible to receive up to $610-million in upfront fees and milestones for all three targets, as well as research funding and tiered royalties on sales.
How would you describe your business model?
We think of ourselves as a pure play technology company. We share in the upside with our partners in terms of milestones and royalties, but they pay for the development work and we avoid the binary risk that a biotech company takes. This allows us to expand the number of programs each year. Essentially we are accumulating a diversified portfolio of next-generation drugs that we are creating with our partners. We value ourselves based on the net present value of our cumulative programs over time.
In the future, we may selectively carry our own internal drug candidates through to preclinical or IND stage and out-license the candidates for clinical advancement by a partner for a potentially greater share in the economics. This will keep us laser focused on the partnership model and let us play to our strength of drug discovery, leveraging our partners’ expertise in biology, clinical trials, and commercialization.
Where do you envision Absci will be in five years?
Absci is working towards a fully in silico drug creation AI pipeline that will be able to design the optimal therapeutic protein for any target, or for any patient, as well as select the appropriate solutions for its production cell, with the click of a button before stepping into the lab. I think in five years we’ll be clicking a lot of buttons to generate a lot of great drug candidates. With our synthetic biology platform strengths, I’d like Absci to be the go-to provider of technology for generating next generation biologics so when a potential partner discovers a new target, they’ll come to us to help them predict the best drug candidate and associated cell lines and to be able to dramatically accelerate them into the clinic and increase their overall success rate.
• • • • •
To connect with Absci or any of the other companies featured on BioTuesdays, send us an email at [email protected].