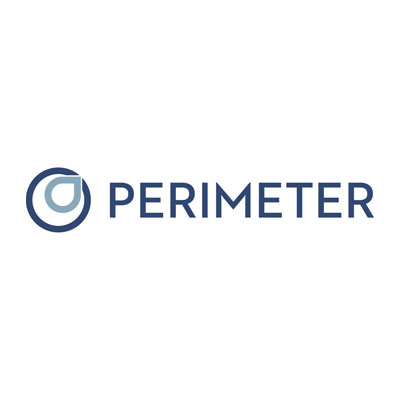
The May issue of Radiology Today magazine features an interview with Andrew Berkeley, co-founder and chief innovation officer of Perimeter Medical Imaging AI (TSXV:PINK; OTCQX:PYNKF), and highlights both the company’s current S-Series OCT and its next-generation B-Series OCT with ImgAssist AI 2.0.
The article, titled An Eye for Detail, outlines that optical coherence tomography (OCT) uses light to create cross-sectional, 3D images of tissue microstructures—delivering10 times the resolution of ultrasound and X-ray, and 100 times the imaging power of MRI. Mr. Berkeley describesOCT as like ultrasound but using a specific wavelength of light instead of sound to capture tissue detail.
Currently, Perimeter is commercializing its first FDA-cleared intraoperative imaging technology, the S-Series OCT, which provides surgeons with image resolutions capable of visualizing tissue structures at the cellular level down to the critical 2mm depth when assessing margins real-time in the OR.
The company’s goal has been to seed the market with S-Series OCT in order to create a strong network of early adopters and technology champions in preparation for a potential FDA clearance of its next-generation system, B-Series OCT with ImgAssist AI 2.0.
Perimeter B-Series aims to help surgeons determinewhether there is a 2 mm margin of healthy tissue around aremoved breast cancer tumor. Earlier this month, detailed results from the pivotal trial evaluating the use of Perimeter B-Series for intraoperative margin assessment during breast-conserving surgeries were presented during the scientific session of the 26th Annual Meeting of the American Society of Breast Surgeons (ASBrS) by the trial’s Primary Principal Investigator, Dr. Alastair Thompson. As previously announced, the pivotal trial met its primary endpoint with statistically significant reduction in patients with residual cancer during surgery (p-value = 0.0050) and demonstrated super-superiority compared to standard-of-care alone. Among other positive findings, Dr. Thompson’s ASBrS presentation highlighted that use of Perimeter B-Series has the potential to change the current paradigm by empowering surgeons to identify regions of interest, enhance real-time intraoperative decision-making, and reduce the incidence of re-excision due to unaddressed residual disease following lumpectomy.
In March 2025, Perimeter announced the submission of a Premarket Approval (PMA) application to the FDA for use of Perimeter B-Series during breast cancer surgeries in the U.S. The FDA PMA submission represented the achievement of a major milestone – Perimeter’s first pre-market regulatory submission for its AI-enabled, wide-field OCT technology, as well as for a specific indication label. The FDA PMA application, supported by recent pivotal trial results, is currently under review by the Agency.
Beyond breast cancer, the article notes that Perimeter is also exploring OCT for use in head and neck surgeries—for example in the treatment of oral cancer. “There’s a big sensitivity about taking too much tissue in the oral cavity, so surgeons are always trying to spare, as you can imagine, tissue around the oral cavity,” Mr. Berkeley said.
OCT enables real-time, intraoperative imaging of tumors and provides guidance on margin status. “Even though there is less volume of head and neck cancer cases, the impact of reducing positive margins is a lot greater,” he added. Positive margin implications for head and neck cancers have significant negative impact on patient mortality rates.
The article highlights that Perimeter has developed machine learning algorithms to analyze OCT images, identifying areas of interest and helping surgeons interpret the scans more effectively. The company is also using AI to accelerate image acquisition through interpolationtechniques. “It’s very difficult to accumulate all of that data when you’re in the early stages because you don’t have broad adoption of your technology, but we’re in the process of building these datasets. So, we’re also looking at ways of augmenting existing data, for example we pick one good quality AI training label and then augment that in many different ways so that now one label turns into 10 or 15 labels,” Mr. Berkeley said.